Table Of Content

For instance, relative to some complex interactions, main effects are more easily interpreted (Collins et al., 2014); a factor’s main effects are interpretable even when it interacts with other factors. When effect coding is used, each effect is orthogonal to every other effect in the analysis model (orthogonal when the n’s are equal in each experimental condition, and nearly orthogonal when the n’s differ by a modest amount). Thus, a significant main effect reflects an experimental effect that occurs on average across all other factors in the model even when the relevant factor is involved in significant interactions (Chakraborty et al., 2009).
2: Design of experiments via factorial designs
For instance, RCTs need not have a true placebo or control condition. Thus, two different active treatments might be contrasted with one another in a two-group design, such as a comparison of two different counseling approaches (e.g., skill training vs. supportive counseling), each paired with the same medication. Neither one of these conditions would be a control condition in a strict sense, since each delivers a different form of active treatment. In addition, an RCT might have a control condition, but this might be used in comparisons with many active treatment conditions.
1: Factorial Designs
Next, what we really want to look at is the factorial plots for these three factors, B, C and D and the interactions among these, BD and BC. The first is to take a higher order interaction out of the model and use them as the estimate of error. Next, what we did at the end of the process is drop that factor entirely. If a particular factor in the screening experiment turns out to be not important either as a main effect or as part of any interaction we can remove it. This is the second strategy, and for instance in this example we took out factor B completely from the analysis.
1.4. Measures of the Same Construct¶
The material found on this site is for informational purposes only and is not intended to be used for diagnosis or treatment purposes. So, the researchers decided to look at how the amount of sleep and caffeine influence test performance. “It is true that when two manipulations are operating simultaneously, it is impossible to disentangle their effects completely,” explain authors Breckler, Olson, and Wiggins in their book Social Psychology Alive.
4. Complex Correlational Designs¶
We can look at this two ways, and either way shows the presence of the very same interaction. First, does the effect of being tired depend on the levels of the time since last meal? Look first at the effect of being tired only for the “1 hour condition”. We see the red bar (tired) is 1 unit lower than the green bar (not tired). So, there is an effect of 1 unit of being tired in the 1 hour condition. Next, look at the effect of being tired only for the “5 hour” condition.
This is because, as noted earlier, such effects are determined by averaging over the other component effects (with effect coding). In addition, the complexity of delivering multiple combinations of components can be reduced by using a fractional factorial design (Collins et al., 2009), which reduces the number of different component combinations per the number of factors used. While more research on IC interactions is surely needed, our research has consistently found such interactions (Cook et al., 2016; Fraser et al., 2014; Piper et al., 2016; Schlam et al., 2016). Thus, it might be difficult in many cases to assume conditions that would justify the use of a fractional factorial design.
Figure 5.3 shows results for two hypothetical factorial experiments. Time of day (day vs. night) is represented by different locations on the x-axis, and cell phone use (no vs. yes) is represented by different-colored bars. It would also be possible to represent cell phone use on the x-axis and time of day as different-colored bars.
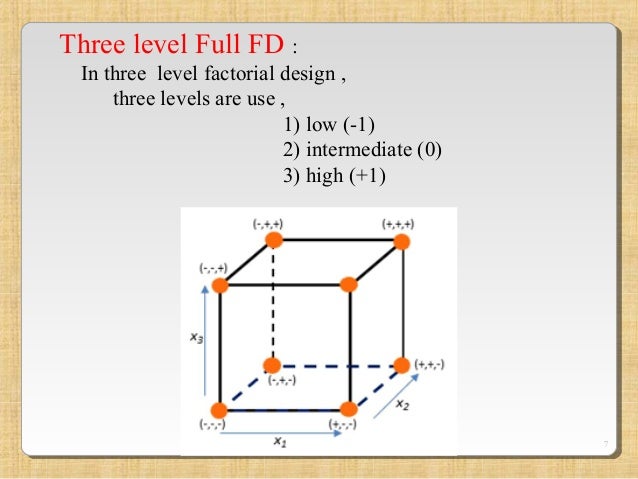
Contrasts, main effects and interactions
EFSA: Poor Hygienic Equipment Design Most Crucial Risk Factor for Persistent Pathogens in Food Production - Food Safety Magazine
EFSA: Poor Hygienic Equipment Design Most Crucial Risk Factor for Persistent Pathogens in Food Production.
Posted: Tue, 23 Jan 2024 08:00:00 GMT [source]
A 2×2 factorial design is a type of experimental design that allows researchers to understand the effects of two independent variables (each with two levels) on a single dependent variable. There is an interaction effect (or just “interaction”) when the effect of one independent variable depends on the level of another. Although this might seem complicated, you already have an intuitive understanding of interactions.
A 2 means that the independent variable has two levels, a 3 means that the independent variable has three levels, a 4 means it has four levels, etc. To illustrate, a 3 x 3 design has two independent variables, each with three levels, while a 2 x 2 x 2 design has three independent variables, each with two levels. In general, when dummy coding is used, the effects corresponding to main effects in a standard ANOVA are similar to simple effects, i.e., the effect of a variable when all other variables in the model are set to the level coded as zero. For instance, in the design depicted in Table 1, the effect of Extended Medication would be reflected by the average effect of all Extended Medication conditions (1–16) versus the average effect of all Standard Medication conditions (17–32).
However, by choosing B at the low level you will produce a more homogeneous product, products with less variability. What is important in product manufacturing is not only reducing the number of defects but also producing products that are uniform. This is a secondary consideration that should be taken into account after the primary considerations related to the percent of product defects. For each factor, you see that the residuals are more dispersed (higher variance) to the right than to the left. Overall, however, the residuals do not look too bad and the normal plot also does not look too bad.
Distributive randomization: a pragmatic fractional factorial design to screen or evaluate multiple simultaneous ... - BMC Medical Research Methodology
Distributive randomization: a pragmatic fractional factorial design to screen or evaluate multiple simultaneous ....
Posted: Mon, 11 Mar 2024 07:00:00 GMT [source]
Investigators should consider using measures that would be sensitive to such effects. For instance, investigators might assess measures of burden (treatment fatigue) and determine if these are especially highly related to particular ICs or to an increasing number of ICs. Indeed, even without the use of special assessments, investigators might correlate the number of ICs a person receives (regardless of type) to outcomes. This table reflects the combinations of intervention components (conditions) that is generated by the crossing of two levels of five factors in a factorial design (Schlam et al. 2016). Interactions occur when the effect of an independent variable depends on the levels of the other independent variable. As we discussed above, some independent variables are independent from one another and will not produce interactions.
Recall that in a between-subjects single factor design, each participant is tested in only one condition. Just as including multiple dependent variables in the same experiment allows one to answer more research questions, so too does including multiple independent variables in the same experiment. For example, instead of conducting one study on the effect of disgust on moral judgment and another on the effect of private body consciousness on moral judgment, Schnall and colleagues were able to conduct one study that addressed both variables. For example, a researcher might choose to treat cell phone use as a within-subjects factor by testing the same participants both while using a cell phone and while not using a cell phone (while counterbalancing the order of these two conditions). An example is a study by Halle Brown and colleagues in which participants were exposed to several words that they were later asked to recall (Brown, Kosslyn, Delamater, Fama, & Barsky, 1999)[1]. Some were negative health-related words (e.g., tumor, coronary), and others were not health related (e.g., election, geometry).
The advantage of this is that multiple-response measures are generally more reliable than single-response measures. However, it is important to make sure the individual dependent variables are correlated with each other by computing an internal consistency measure such as Cronbach’s \(\alpha\). If they are not correlated with each other, then it does not make sense to combine them into a measure of a single construct. If they have poor internal consistency, then they should be treated as separate dependent variables. A factorial experiment allows for estimation of experimental error in two ways. The experiment can be replicated, or the sparsity-of-effects principle can often be exploited.
No comments:
Post a Comment